Customer story
Cash flow forecasting
Karl Mayer
An international group of companies with deep roots in the textile machine building sector

Year founded
1937
Industry
Machinery manufacturing
About
KARL MAYER is an international group of companies with deep roots in the textile machine building sector. The group offers solutions for knitwear factories, technical textiles, warp preparation, flat knitting and digital solutions.
It employs more than 3,300 personnel and has subsidiaries in the US, England, India, Italy, Hong Kong, Japan, China, Bangladesh and Switzerland, as well as sales offices all around the globe.
Challenge
Operating at the leading edge of economic development, the first impacts of the oncoming pandemic were already being felt in spring 2020. The globally challenging environment in terms of incoming orders meant that the Group already felt the need to reorganize its planning and forecasting processes to achieve more visibility in the short term, make the process more efficient and significantly more accurate.
Solution
Against this backdrop, KARL MAYER decided to largely automate its cash flow forecasting in order to reduce the need for human resources and to be able to respond better to country-specific challenges and restrictions with Nomentia's AI-powered Cash Flow Forecasting software solution.
“As a B2B business group, we knew that we were taking a certain risk, but Nomentia was transparent about this from Day 1 and we weren’t disappointed. Thanks to the predictive analytics feature in Nomentia, we feel that we are being ideally supported in the current economic environment, and that is a good feeling.”
Sven Erik Ebel, Vice President Treasury and Credit Management, KARL MAYER
Statistical methods are applied by many software providers these days. However, these often underestimate the results can only be as accurate as the underlying assumptions, which vary depending on the business model and the client. For B2B corporates with predominantly irregular payments, implementing a high-quality, predictive forecast has in the past been considered unachievable with chances of success even smaller in a period when a global pandemic upturns all the figures. Nonetheless, the KARL MAYER Group and Nomentia took the plunge and are extremely satisfied with the results.
Fig. 1 | Patterns in the past provide an insight into the future: A very simplified explanation of predictive analytics.
But is predictive analytics the right approach for a B2B corporate? This is the question that a pilot project aimed to answer. Sven Erik Ebel, Vice President Treasury and Credit Management at KARL MAYER, highlights the company’s willingness to innovate: “Our corporate group works innovatively in all business areas. We even have a business unit dedicated solely to digitalization issues. Predictive analytics was therefore not an alien concept for us but something already being used in other departments. That made of curious to find out whether this process could also be used profitably in treasury.”
Tricky starting point: mainly one-off B2B payments and a global corona crisis
However, not all predictive analytics is really predictive analytics. When it comes to cash flow forecasting: Historical cash flow data spanning a period of at least three years are the most important ingredients for accurate predictions. These data are analysed for trends and patterns using a statistical computation model and projected into the future while also taking external factors, such as economic indicators or market indices, into account. For this to work, the calculation model needs to be configured in advance so that it can categorise recurring events (e.g. the impact of public holidays) and ignore data outliers.
Fig. 2 | Factors influencing companies’ cash flow developments
Put very simply, this explains why predictive analytics can only deliver accurate results if a suitable data basis exists. Both the impacts of the pandemic and the one-off nature of the projects meant even at the outset of the project that it would be very challenging to identify applicable patterns in the historical data of this machine builder and then be able to sufficiently calibrate the models used.
KARL MAYER wouldn’t however be the global market leader in many segments if such challenges were enough to dampen its innovative spirit. “The pandemic was a huge stress test for us all. That’s why there was no reason not to tackle it with the latest methods,” explains Sven Erik Ebel.
A client all software providers would love to have. In April, the data scientists from Nomentia were given access to all existing historical data from prior financial years and immediately started their analysis. Together with the treasury team, it was determined which data and influencing factors were relevant for the forecasts. The textile manufacturing index was seen here to be especially important, but seasonality and trends were also considered during the project.
Fig. 3 | Test run with historical data over a period of three years and analysis of the best calculation model: The first two years of actual data are used to validate the computation model. The model which most closely reflects the results in the third year (red chart) is then selected as the basis for calculating the forecasting data. At least three years should be available; ideally four.
The analysis presented the team with the anticipated challenges
The existing data soon made it clear that the one-off nature of the projects mentioned above lead to fluctuations in cash flow developments of up to 400 percent – a major stress test for project implementation. The data scientists and the treasury team at KARL MAYER had to manually interpret the outliers for the system and also ask themselves how these figures could be differentiated from regular phenomena such as payment runs, seasonality and the like.
The data analysis was completed at the end of April and yielded a pre-selection of five statistical models for the first test run. The next step entailed the treasury software Nomentia calibrating the models using the previously identified influencing factors and calculating a testing plan for the past twelve months based on the actual historical data.
The outcome of the first test run was sobering and highlighted considerable need for optimisation. The ‘R Standard Package’ used to correct outliers was not suitable for identifying outliers and, in particular, correcting these to a defined value.
Fig. 4 | An example of outliers: The red line indicates that January was the best month for customer payments in 2020. In all three previous years, however, January had been among the worst months. Without taking these effects into consideration, a prediction would also deliver a far worse forecast.
The solution: rethink!
Johannes Pöschl, data scientist at Nomentia, on this issue: Although ‘special’ project-related figures can be designated as such by the client, this means a lot of work in the case of a volatile project-based business segment and doesn’t clarify what needs to be corrected given that outliers are not one-time (and therefore easily eliminated) special payments but are dependent on a project portfolio consisting of major-specific projects. The aim however is to automate the forecasting, which is why we couldn’t be entirely satisfied with just manual corrections.”
The next step involved Pöschl and his team deftly developing a new method, which after having been thoroughly tested prior to deployment - could perform specific outlier corrections for KARL MAYER taking seasonality, long-term trend developments and external effects (such as COVID-19) into account. The precise approach is something that the team wants to keep under wraps for good reason and would have been too complex to explain in this article.
A further step entailed eliminating unsuitable models from the calculations in advance, so as to deliver clearer results. Besides this, the calculations were repeated using various model pools to subsequently compare the most successful models with each other and to ultimately select the best-fitting one. By the end of May, all the preconditions had been met for preparing a group-wide cash flow forecast.
Fig. 5 | Statistical model groups that Nomentia uses in predictive analytics: There is nearly no end to the statistical models available but not all are suitable for cash flow forecasting. Nomentia relies on the following eight model groups
Mission accomplished – the KARL MAYER Group now calculates its monthly forecasts largely automatically
In combination with the specific expertise of the treasury team members, the forecasting data generated now form a sound basis for the treasury department at the KARL MAYER Group, to swiftly prepare professional cash flow forecasts. For some forecasting categories (e.g. salaries), this works so reliably that Nomentia can even directly generate a cash flow forecast for the group if wanted. For categories which are often dominated by exceptions, Nomentia displays the calculated figures in the standard forecasting screen as proposals. In this case, the existing data act as a guide for manual fine-tuning and save valuable time.
Fig. 6 | Manual forecasting proposals: Predictive analytics provides a baseline which can then be easily adjusted manually.
The results are impressive: The forecasts generated automatically are more accurate than the previous manual forecasts while taking less time. Among other reasons, this is because predictive analytics performs assessments without relying on subjective background knowledge, which is often a major advantage. Although this rarely applies in the case of short-term predictions, long-term trends over a period of six months or a year deliver results which, with an accuracy rate of 94.5%, are amazingly similar to the real data.
Sven Erik Ebel: “Perfect forecasting at the press of a button is illusionary; that is clear. But predictive analytics must make the work easier. It needs to be at least as reliable as a manual forecast and it must of course also save time. One of our subsidiaries which requires very complex forecasting, which cost us a lot of time in the past, has even increased the accuracy of its forecasting by three percent due to predictive analytics. At first, that might not sound like a big difference but, if you consider the massive time-savings, this is a great result for us.”
An exciting project and one which has significantly advanced the usability of predictive analytics and shown that treading new paths can pay off. Even, and perhaps especially, during challenging periods.
Improved treasury management starts with Nomentia
Would you like to discover how we can assist your treasury team?
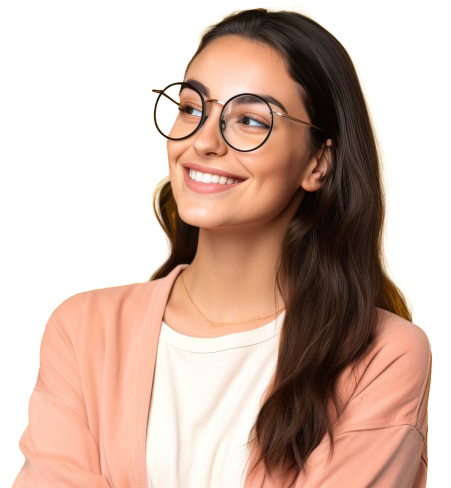