Your cash flow forecast is lying to you. Not on purpose, but it’s wrong—probably by a lot. Spreadsheets lull you into a false sense of control, but the numbers lie. They miss shifts in spending, overlook delayed payments, and crumble the moment reality deviates from last month’s assumptions. By the time the cracks show, you’re already scrambling—plugging holes, chasing down cash, and making desperate decisions.
The experts:
Hubert Rappold
Hubert Rappold works as a Senior Treasury Expert at Nomentia, bringing his over 20 years of expertise to serving Nomentia's customers' treasury needs and challenges in payments, cash visibility, and forecasting.
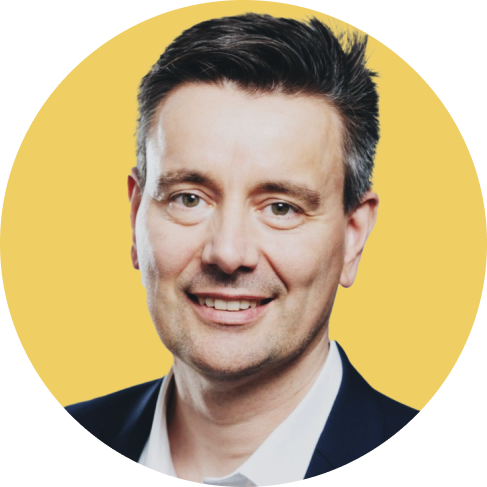
Johannes Pöschl
Johannes Pöschl is a Senior Data Scientist at Nomentia, specializing in predictive analytics and AI-driven cash flow forecasting solutions.
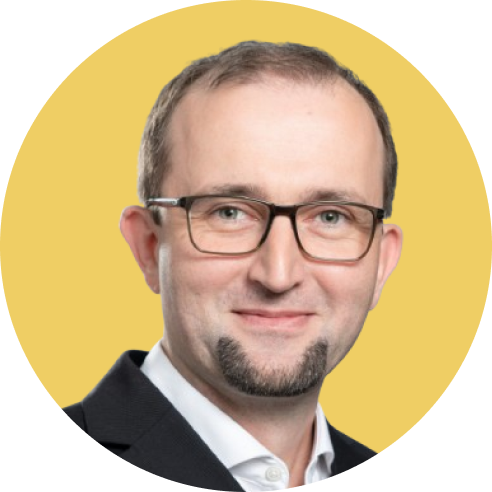
Why "close enough" isn’t always good enough in cash flow forecasting
A cash flow forecast that’s even slightly off can send your business into a tailspin. As Hubert Rappold puts it: “Small deviations in cash flow forecasts can lead to big financial risks.” Underestimate expenses, and you’re suddenly short on cash when payroll hits. Overestimate revenue, and you’re making spending decisions based on money that isn’t actually there. "Basing your decision on a forecast that is substantially off; you might end up making decisions based on inaccurate data—like borrowing unnecessarily or mismanaging liquidity.” The further your forecast drifts from reality, the riskier every financial move becomes. And in a world where margins are tight, customers pay late and surprises lurk around every corner; imprecise forecasting isn't just an inconvenience but a liability.
What is cash flow forecasting, really?
At its core, cash flow forecasting is just this: predicting how much money will move in and out of your business over a given period. It’s not about spreadsheets or fancy formulas but knowing, with as much certainty as possible, whether you’ll have enough cash to cover what’s coming.
Why traditional forecasting falls apart?
Most businesses, big and small, still rely on outdated tools and methods that weren’t built for today’s financial landscape. Here’s why they fail:
- Excel is static – Spreadsheets don’t update themselves. Every forecast relies on manual inputs, meaning errors, omissions, and outdated data pile up fast.
- Assumptions age poorly – Traditional models rely too much on past data, ignoring real-time shifts like late payments, economic changes, or unexpected expenses.
- It’s a time sink – Forecasting manually eats up hours (or days), and by the time you're done, the numbers may already be obsolete.
- Human error is inevitable – Even the best finance teams make mistakes. One wrong formula or missing invoice can throw everything off.
- No real-time adjustments – A single unexpected expense or delayed payment can make a forecast useless, but Excel won’t flag the problem until it's too late.
- Data lives in silos – Bank accounts, invoices, and ERPs all hold key pieces of the puzzle, but pulling them together manually is slow, painful, and prone to mistakes.
- Key person dependency – Many companies rely on one finance expert who "knows the spreadsheet." If they leave, get sick, or make a mistake, the entire system can fall apart.
Let’s be clear—treasurers and finance managers are some of the most skilled problem-solvers in any business. They know how to fine-tune their forecasts, clean up messy data, and make Excel do what they need. But time is limited, and expectations keep rising. The demand for instant, up-to-date cash visibility means they can only do so much, no matter how skilled they are.
"Excel-based forecasting worked perfectly fine when business environments were more predictable," explains Johannes Pöschl. "But today, cash flows are influenced by a web of factors—seasonality, economic shifts, even supplier behavior. Static spreadsheets just can’t keep up with that complexity."
And here’s the real danger: many forecasts depend entirely on the person who built them. If that expert is unavailable—whether they leave, take a vacation, or simply get swamped—no one else knows exactly how their formulas and models work. That’s a terrifying prospect for any company relying on accurate cash flow predictions.
This is where AI-driven automation changes the game. Instead of a fragile, human-dependent system, businesses get a dynamic, always-updating forecast that adjusts in real time. It doesn't replace the finance team—it gives them superpowers. Let’s take a look at:
How AI enhances cash forecasting accuracy?
For many, forecasting cash flow, is a reactive scramble. No wonder, when traditional forecasting relies on static models and best guesses. Implementing AI into your forecasting you can go beyond static formulas and outdated assumptions. Not just automate your forecasting, but make it smarter, spot hidden patterns and continuously refines projections.
"AI forecasting can lead to more objective forecasts, leaving behind the impact of regional optimism biases in forecasting,” says Johannes. “They can also incorporate resource prices and estimate their effects on supplier prices that traditional models or treasurers might miss."
As Hubert adds: “AI can automatically classify transactions from bank statements, showing finance teams exactly where cash flow discrepancies are coming from—late customer payments, unexpected supplier costs, or seasonal trends. That kind of insight is invaluable."
Here’s how it stacks up against traditional methods:
Traditional forecasting methods | Challenges for forecast accuracy | AI in forecasting: Key techniques for accuracy improvement |
Manual data entry & spreadsheets | Prone to human error, delays, and data inconsistencies | Automated connectivity & forecast reconciliation: integrating real-time bank & ERP data to eliminate errors and update forecasts dynamically. |
Rule-based forecasting (fixed models) | Rigid assumptions fail to capture real-world volatility | Machine learning & pattern recognition: AI-powered forecasting learns from past errors and adapts dynamically to new trends. |
Historical trend extrapolation | Fails to account for sudden economic shifts, external shocks | Multi-variable analysis: AI-driven forecasting incorporates economic indicators, market trends, and business-specific variables. |
Limited scenario planning | Forecasts become unreliable in times of uncertainty | Risk simulations & stress tests: AI forecasting can run multiple scenarios to assess financial resilience under various conditions. |
Static payment terms-based Cash flow predictions | Overlooks customer-specific behavior, which leads to inaccurate receivables forecasts | Dynamic payment behavior predictions: AI forecasts can analyze past payment trends to predict late payments with higher accuracy. |
Isolated departmental forecasting | Fragmented cash flow data across finance, treasury, and operations | Automated connectivity: Forecasts integrate multiple data sources for a holistic, real-time view of liquidity. |
Lack of external market consideration | Ignores macroeconomic trends, FX rates, inflation, and geopolitical risks | Sentiment & market trend analysis: AI-supported forecasts process can incorporate market sentiment indices, GDP forecasts, interest rates and other data affecting the business environment to refine forecasts. |
Reactive forecast adjustments | Adjustments are made after discrepancies occur, not proactively | Self-learning algorithms: continuous refinement of forecasts based on real-time variance analysis. |
Delayed cash flow reconciliation | Forecasts deviate from reality due to mismatches in receivables/payables data. | Automated invoice matching & forecast reconciliation: reconciling forecasts against actual bank transactions in real-time. |
AI in cash forecasting: Use case examples
"Take something as simple as public holidays," says Johannes. "They affect cash flows differently depending on the industry and country, and AI can model these effects automatically. Over time, the system refines itself, making forecasts even more accurate."
Hubert gives another example: "AI can analyze past customer payment behaviors to refine expected due dates. But even simple logic—like applying Days Sales Outstanding (DSO) metrics—can significantly improve accuracy, especially for short-term forecasts."
Let’s consider:
- Pattern recognition to catch supplier payment delays: An industrial equipment manufacturer kept running into unexpected Q4 cash shortfalls. The culprit? Subsidiaries in Asia were consistently paying suppliers 15–20 days late, but traditional models failed to flag the pattern. The result: a €10M gap, covered by borrowing at 5% interest—wasting €125K per quarter. AI-powered forecasting caught the recurring delays, allowing the treasury to adjust projections and secure credit lines early, saving €500K a year.
- Predicting late payments to stop cash flow gaps: A software company with €50M in receivables struggled with late payments from enterprise clients in North America and Asia. Customers paid an average of 12 days late, forcing treasury to rely on credit lines at 4% interest—burning €65K every month. Using AI to analyze payment behaviors, allowed the treasury to predict which clients would delay, and helped them follow up proactively. Cutting late payments by half saved €390K per year in financing costs.
- Market sentiment analysis to see a demand drop before it happens: An automotive parts supplier was blindsided by a slowdown in US and UK car manufacturing. Depending on traditional forecasting would have allowed them to miss the warning signs, leaving the company stuck with €30M in excess inventory—costing €3M per year in storage. Perhaps, the right person at the right place at the right time would have caught the demand drop and acted accordingly, but they weren’t available. AI assistance, however, had allowed the treasury to detect a downturn in auto sales three months in advance. Because the treasury acted early, they were able to reduce inventory buildup and avoid €1.4M in storage and emergency financing costs.
The Treasurer’s dilemma: Drowning in Spreadsheets, starved for time
Every morning, the Treasurer of a globally operating business opened the same monster Excel file—a tangled web of formulas, manual inputs, and linked sheets that somehow held the key to the company’s cash flow. Keeping it updated was a full-time job. Data trickled in from subsidiaries across time zones, bank accounts were scattered across multiple institutions, and assumptions had to be constantly tweaked. Forecasting was supposed to provide clarity, but instead, it felt like a high-stakes guessing game.
The demands from leadership kept growing: More accuracy. More real-time visibility. More risk mitigation. But with what? The Treasurer had already pushed Excel to its limits, building an intricate system that only they truly understood. When the CFO needed answers, they delivered—but not without late nights, countless emails chasing missing numbers, and a nagging fear that one small mistake could throw everything off.
Then came the talk about AI-powered forecasting.
It sounded promising—automation, real-time data analysis, better predictions. But there was also an unspoken worry: What if this replaces me? What if all my expertise, my hard-earned knowledge, gets sidelined by software?
And yet, the bigger fear wasn’t AI. It was this. This endless cycle of manual work, desperate fixes, and hoping that when leadership asked for insights, the numbers weren’t off—because if they were, it would be their name on the line. Worse still, if they ever stepped away, who else would even know how to keep this monster running?
That’s when the real question hit: What’s the bigger risk—adopting AI or continuing like this? Spending hours babysitting spreadsheets?
The reality hit. So they ran the numbers. Even a small boost in forecast accuracy would cut emergency borrowing and save more than enough to justify the investment. The business case was clear. It was time to kill the spreadsheet before the spreadsheet killed them.
"A lot of treasurers worry that AI will replace them," Johannes notes. "But in reality, AI is just a tool—it provides forecasts, but treasurers still bring the expertise to validate and interpret them. The most successful teams use AI to eliminate tedious manual work, freeing themselves to focus on strategy."
Hubert agrees: "If you set up AI-driven forecasting right, you don't just improve accuracy—you make life easier. Treasurers get instant feedback, can compare past forecasts to actuals, and refine their approach over time. The goal isn't to replace them; it's to give them better tools."
Conclusion: Forecast or guess?
You wouldn’t steer a company based on gut feelings alone—so why accept guesswork in cash flow forecasting? AI isn’t a magic fix, but it’s the difference between informed decisions and financial blind spots. The real risk isn’t AI—it’s sticking to spreadsheets while the world moves forward.